Efficient Computing Lab.
The Efficient Computing Laboratory (ECL) is a part of Department of AI at UST ETRI Campus. Gajeong-ro 218, Yuseong-gu, Daejoen South Korea.
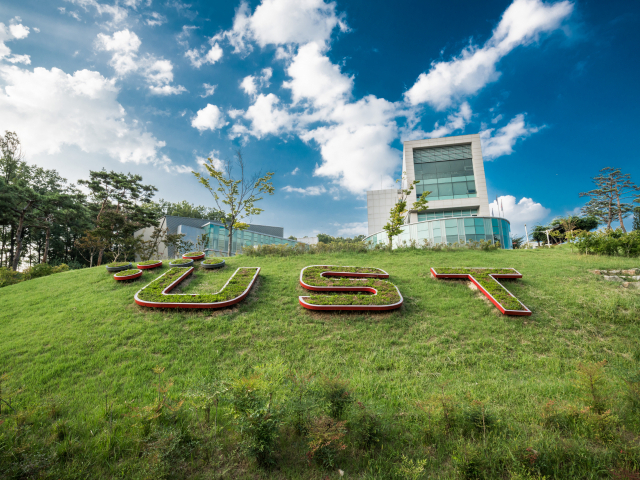
7-416 ETRI, Gajeong-ro 218,Yuseong-gu123, Daejeon, South Korea
Welcome to the Efficient Computing Lab.
We focus on energy efficiency, system optimization, user experience, and sustainable tech solutions. Our research interests are the followings:
• Model Compression: Enhancing machine learning models’ efficiency through techniques like pruning, quantization, and knowledge distillation for better performance in resource-limited settings.
• AI Compiler: Developing optimized AI compilers to reduce computational power, energy use, and execution time, improving efficiency and sustainability.
For detailed research areas and insights into graduate life, please refer to the following slides. Students interested in pursuing graduate studies are encouraged to contact me directly after following the application instructions provided in the slides.
You can reach me at: leejaymin_at_etri_dot_re_dot_kr.
News
Feb 21, 2025 | Our paper was accepted at Sensors Journal (IF: 3.4, JCR24 Top 30.92%, Q2)![]() |
---|---|
Dec 17, 2024 | Our paper was selected as a Distinguished Paper in the 1st International Conference on Artificial Intelligence Computing and Systems (AICompS) 2024![]() |
Dec 15, 2024 | “QuantuneV2” was accepted at Future Generation Computer Systems Journal (IF: 6.2, JCR23 Top 9.4%, Q1)![]() |
Nov 14, 2024 | Our paper received Best Paper Award in 2024 IeMeK, held at Jeju in 13–16 Nov. 2024![]() |
Nov 8, 2024 | Two papers was accepted at AIcompS 2024 . Congratulations![]() |
Oct 11, 2024 | ML^2Tuner paper was accepted at NeurIPS 2024 Workshop on ML for Systems . Congratulations![]() |
Aug 19, 2024 | Mixed Non-linear quantization paper was accepted at ECCV Workshop-CADL . Congratulations![]() |